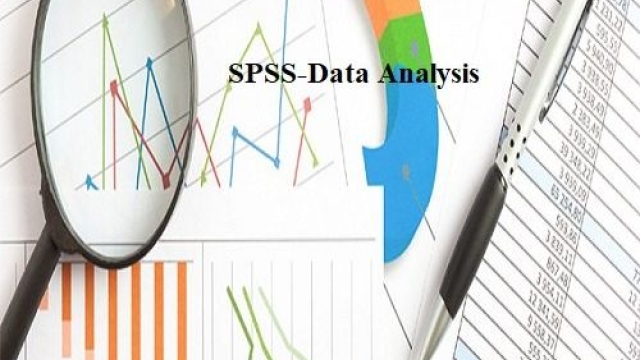
Unlocking Insights: Demystifying Dissertation Data Analysis
As an aspiring academic, one of the most significant milestones in your journey towards obtaining a doctoral degree is completing your dissertation or capstone project. These comprehensive research endeavors require meticulous planning, dedication, and persistence. Once you’ve collected all the necessary data, a critical phase awaits you: dissertation data analysis.
Data analysis plays a vital role in uncovering insights and drawing meaningful conclusions from the vast amount of information you’ve gathered. It involves organizing, interpreting, and synthesizing your data to identify patterns, make connections, and support your research hypotheses. However, this phase of the dissertation process can often be intimidating, as it requires a solid understanding of statistical techniques and data analysis tools.
In this article, we will demystify the world of dissertation data analysis, guiding you through the essential concepts, strategies, and tools you need to unlock the insights hidden within your data. Whether you are embarking on a qualitative or quantitative study, we will explore various data analysis methodologies and provide practical tips to help you navigate this challenging terrain. By the end of this article, you will be better equipped to tackle your dissertation data analysis with confidence and precision. So let’s dive in and unravel the mysteries of data analysis in the context of dissertations and capstone projects.
Importance of Data Analysis in Dissertations
In the realm of dissertations and capstones, the process of data analysis holds paramount significance. It serves as the backbone for drawing meaningful conclusions and uncovering valuable insights from the gathered information. Without a comprehensive data analysis, the research findings would remain incomplete and lack the substantiation required for academic rigor.
Data analysis in dissertations allows researchers to make sense of the intricate details and patterns hidden within the collected data. By employing various statistical techniques and tools, researchers can unravel the underlying trends, relationships, and correlations that are crucial for contributing to existing knowledge and theory. This process aids in identifying significant findings and validating the research hypothesis, thereby enhancing the overall credibility and academic value of the study.
Furthermore, data analysis in dissertations facilitates the identification of outliers, anomalies, and potential limitations within the research. It helps researchers understand the scope and boundaries of their study, enabling them to address any bias or confounding factors that might impact the validity of the findings. Through meticulous data analysis, researchers can ensure the accuracy and reliability of their results, fostering confidence among the academic community.
Moreover, data analysis offers an avenue for exploring new research avenues and avenues for future inquiry. By delving deep into the data, researchers can identify emerging patterns, identify gaps in current knowledge, and generate novel research questions. This process contributes to the expansion of the academic field by encouraging further investigations and promoting advancements in theory and practice.
In conclusion, the importance of data analysis in dissertations cannot be overstated. It plays a vital role in substantiating research findings, validating hypotheses, addressing limitations, and providing a platform for future research. Through meticulous data analysis, researchers can unlock powerful insights and contribute to the growth of knowledge within their respective fields.
Key Methods and Approaches for Dissertation Data Analysis
MBA dissertation help
Data analysis in dissertations and capstones plays a crucial role in unraveling meaningful insights. With the aim to demystify the process, this section presents key methods and approaches that are commonly utilized in dissertation data analysis.
Quantitative Analysis:
Quantitative analysis involves the examination of numerical data to identify patterns, relationships, and trends. It employs statistical techniques such as regression analysis, correlation analysis, and hypothesis testing to draw conclusions and make generalizations based on a representative sample. By applying mathematical models, researchers can quantify and measure variables, enabling them to explore the impact of different factors on their research questions.Qualitative Analysis:
Qualitative analysis focuses on understanding and interpreting non-numerical data, including interviews, observations, focus groups, and textual documents. Researchers using this approach engage in a systematic coding and categorization process to identify common themes, ideas, and patterns emerging from the data. Through qualitative analysis, they aim to gain a deeper understanding of the lived experiences, perspectives, and contextual nuances surrounding their research topic.Mixed-Methods Analysis:
A mixed-methods approach combines both quantitative and qualitative analysis techniques to gain a comprehensive understanding of the research problem. By integrating the strengths of both approaches, researchers can triangulate their findings, validate results, and potentially uncover new insights. This method enables the researcher to explore complex research questions by drawing on the benefits of both numerical and non-numerical data.
In dissertation data analysis, researchers often adopt one or a combination of these methods, depending on the nature of their research questions and the availability of relevant data. It is crucial to carefully select and align the chosen methods with the research objectives to ensure accurate and meaningful interpretations of the collected data.
Tips and Recommendations for Effective Dissertation Data Analysis
To ensure a successful dissertation data analysis, consider the following tips and recommendations:
Choose the Right Analytical Techniques: Selecting the appropriate analytical techniques is crucial for extracting meaningful insights from your dissertation data. Make sure to thoroughly understand the nature of your research questions and data before deciding on the most suitable statistical methods or software to employ. By aligning your analytical techniques with your research objectives, you can enhance the accuracy and reliability of your findings.
Ensure Data Quality and Preprocessing: Before diving into the analysis phase, it is essential to ensure the quality and integrity of your data. Take time to clean and preprocess your data by identifying and resolving any missing values, outliers, or inconsistencies. This process will help you eliminate any potential biases or confounding factors that could impact the reliability of your analysis. Remember, the quality of your results is only as good as the quality of your data.
Document Your Analysis Process: Documenting your data analysis process is a critical aspect of any dissertation study. By keeping a detailed record of the steps you took and choices you made during the analysis, you can enhance transparency, credibility, and replicability. This documentation will also prove invaluable when it comes to discussing and defending your findings with your academic committee or future researchers interested in your work.

Following these tips and recommendations will enable you to conduct effective dissertation data analysis, leading to valuable insights and a successful research outcome. Remember, diligent planning, careful selection of analytical techniques, and thorough documentation are key elements to unlocking the full potential of your dissertation’s data analysis.